by Margaret Warren
The following post introduces to you ImageSnippets and how this tool profits from the use of DBpedia.
ImageSnippets – A Tool for Image Curation
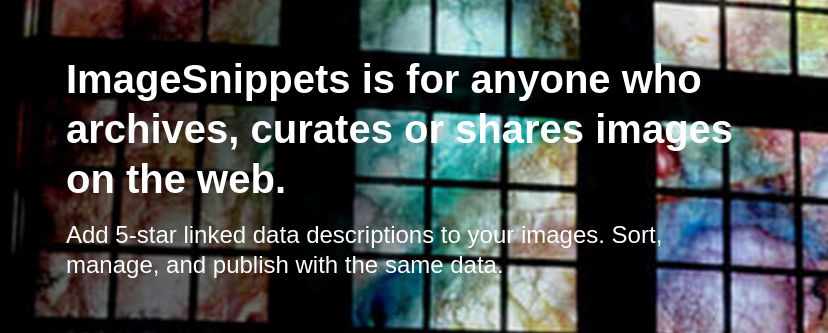
For over two decades, ImageSnippets has been evolving as an ontology and data-driven framework for image annotation research. Representing the informal knowledge people have about the context and provenance of images as RDF/linked data is challenging, but it has also been an enlightening and engaging journey in not only applying formal semantic web theory to building image graphs but also to weave together our interests with what others have been doing in the field of semantic annotation and knowledge graph building over these many years.
DBpedia provides the entities for our RDF descriptions
Since the beginning, we have always made use of DBpedia and other publicly available datasets to provide the entities for use in our RDF descriptions. Though ImageSnippets can be used to build special vocabularies around niche domains, our primary research is around relation ontology building and we prefer to avoid the creation of new entities unless we absolutely can not find them through any other service.
When we first went live with our basic system in 2013, we began hand-building tens of thousands of triples using terms primarily from DBpedia (the core of the linked data cloud.) While there would often be an overlap of terms with other datasets – almost a case of too many choices – we formed a best practice of preferentially using DBpedia terms as often as possible, because they gave us the most utility for reasoning using the SKOS concepts built into the DBpedia service. We have also made extensive use of DBpedia Spotlight for named-entity extraction.
How to combine DBpedia & Wikidata and make it useful for ImageSnippets
But the addition of the Wikidata Query Service over the past 18 months or so has now given us an even more unique challenge: how to work with both! Since DBpedia and Wikidata both have class relationships that we can reason from, we found ourselves in a position to be able to examine both DBpedia and Wikidata in concert with each other through the use of mapping techniques between the two datasets.
How it works: ImageSnippets & DBpedia
When an image is saved, we build inference graphs over results from both DBpedia and Wikidata. These graphs can be revealed with simple SPARQL queries at our endpoint and queries from subclasses, taxons and SKOS concepts can find image results in our custom search tool. We have also just recently added a pathfinder utility – highly useful for semantic explainability as it will return the precise path of connections from an originating source entity to the target entity that was used in our custom image search.
Sometimes a query will produce very unintuitive results, and the pathfinder tool enables us to quickly locate semantic errors which lead to clearly erroneous misclassifications (for example, a search for the Wikidata subclass of ‘communication medium’ reveals images of restaurants and hotels because of misclassifications in Wikidata.) In this way we can quickly troubleshoot the results of queries, using the images as visual cues to explore the accuracy of the semantic modelling in both datasets.
We are very excited with the new directions that we feel can come of our knitting together of the two knowledge graphs through the use of our visual interface and believe there is a great potential for ImageSnippets to serve a more complex role in cleaning and aligning the two datasets, using the images as our guides.
A big thank you to Margaret Warren for providing some insights into her work at ImageSnippets.
Yours,
DBpedia Association
- Did you consider this information as helpful?
- Yep!Not quite ...